Understanding the Data Product Life Cycle
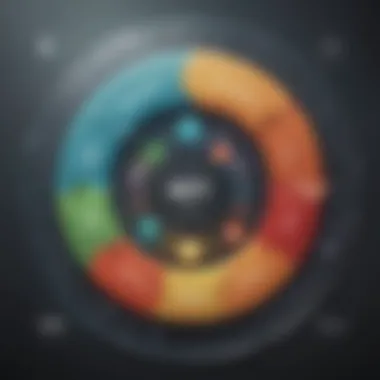
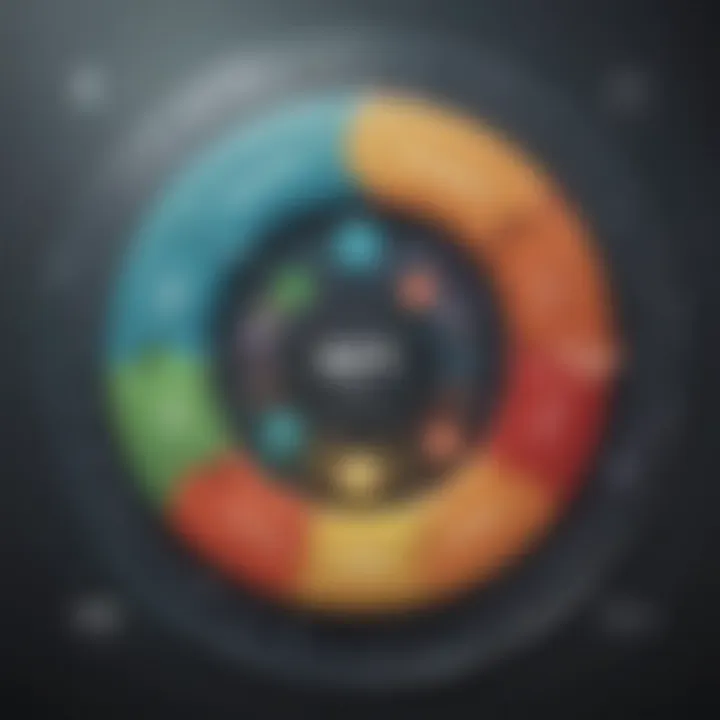
Intro
Data products can take many forms. They can be anything from analytics tools to dashboards or even larger ecosystems that harness raw information into actionable insights. As the data landscape rapidly evolves, comprehending this life cycle equips stakeholders with the insights necessary to adapt and thrive in a competitive environment. This discussion will delve into the nuances of data product development, drawing connections to investment decision-making, which can further enhance the understanding of the financial implications involved.
Investment Dictionaries
Key Terms and Definitions
To lay a solid foundation, it’s essential to clarify some crucial terminology relating to the data product life cycle. Here are a few key terms that often come up in discussions:
- Data Product: A product that utilizes structured or unstructured data to serve a specific purpose, illuminating trends or patterns for its users.
- Lifecycle: The stages through which a data product evolves—from conception to decline.
- Stakeholders: Individuals or groups involved in the data product lifecycle, including data analysts, developers, investors, and users.
- Obsolescence: The stage where a data product is outdated, often due to changes in technology or user needs, leading to its eventual retirement.
These definitions set the groundwork for understanding the intricate dynamics of data product development.
Practical Applications of Investment Terms
When discussing data products, recognizing how investment terminology weaves into the lifecycle is key. Here are some practical applications that investors should mind:
- Due Diligence: Before investing in a data product, stakeholders need to assess the viability and market potential of the product. This phase may involve analyzing the data source, technology stack, and competitive landscape.
- Return on Investment (ROI): Understanding how to calculate ROI for data products can aid investors in determining their financial prospects and sustainability.
- Market Trends: Keeping an eye on market trends related to data products helps investors anticipate shifts in demand, allowing for better portfolio management.
"The key to investing in data products lies in understanding the data not just as numbers, but as stories waiting to be told."
Expert Advice
Strategic Insights for Different Investment Types
When diving deeper into the investment realm associated with data products, various strategies emerge based on the type of investment. For instance:
- Venture Capital: Investors should assess the product's scalability. Evaluating the technology and data models can provide insights into potential for growth.
- Public Equities: Look for companies that consistently innovate their data products to stay relevant within their chosen markets. Historical performance and adaptability should be key indicators.
- Real Estate Investment: In a data-driven era, consider smart cities and how data tech can optimize tenant experiences or administrative efficiencies.
Tips for Evaluating Financial Products
Assessing financial data products involves more than just looking at numbers. Here are some tips to consider:
- Data Quality: Ensure data integrity and reliability. Poor data can lead to flawed insights and, ultimately, poor investment decisions.
- User Engagement: Gauge how well the product resonates with its intended audience. A high user adoption rate often indicates a successful data product.
- Adaptability: Consider how well the product can pivot in response to market changes. Flexibility is often a strong predictor of longevity.
Understanding these elements provides an avenue to navigate the complexities inherent in the data product life cycle. By synthesizing the information presented here, readers can cultivate informed strategies that will serve their investment interests effectively.
Intro to Data Product Life Cycle
The life cycle framework offers a lens through which investors, financial advisors, brokers, and analysts can analyze not only the utility of these products but also their underlying market dynamics. It highlights the necessity for continuous adaptation and improvement, encouraging professionals to anticipate changes and respond accordingly.
The benefits of grasping this life cycle are manifold. First, it allows for better forecasting of product performance. Investors can identify which products are poised for growth or are nearing obsolescence. Furthermore, this knowledge can inform strategies in stakeholder engagement and market positioning, maximizing the chances for successful implementation.
As businesses strive to innovate in an ever-changing environment, managing data products effectively becomes crucial to maintaining a competitive edge. To exemplify this, let’s break down the fundamentals of data products and explore their significance in the broader context of the economy.
Defining Data Products
Data products can be defined as systems or services that utilize data to deliver value to users. They often take the form of dashboards, analytics tools, or application programming interfaces (APIs) that curate and present data in a user-friendly manner. For example, a financial application that compiles stock market data and provides real-time insights into asset performance is a classic data product. By encapsulating data in meaningful formats, these products allow organizations to derive insights and make informed decisions.
What sets data products apart is their reliance not only on data but on the users’ needs. Understanding what users require can direct the design and functionality of the product, making iteratively refined feedback loops paramount.
Importance of the Life Cycle Framework
The life cycle framework serves as a strategic guide, allowing stakeholders to observe and manage data products throughout their existence. Acknowledging the stages—ideation, development, launch, growth, maturity, and decline—enables teams not to just focus on the start but to remain vigilant as conditions shift.
In practical terms, this means:
- Minimizing risks: By being aware of when a product is due for enhancement or possible replacement, stakeholders can mitigate the risk of obsolescence.
- Informed decision-making: Understanding the life cycle of a data product informs investment decisions and the allocation of resources.
- User-centric design: By recognizing how user needs evolve, organizations can adapt their products accordingly, maintaining user engagement across different life stages.
"A well-managed data product life cycle can be the difference between market leadership and a sharp decline."
The implications of this framework extend beyond mere management; they also touch upon ethical considerations and regulatory compliance, two critical aspects in today's data-centric society. As such, a thorough grasp of the data product life cycle is invaluable for navigating the complexities of modern business and investment contexts.
Phase One: Ideation and Conceptualization
The initial phase of the data product life cycle is crucial as it lays down the groundwork for everything that follows. It is the time when ideas take form, and visions become clearer. If this step falters, the entire trajectory may wobble, and later stages could struggle or even derail. The beauty of ideation and conceptualization is that it merges creativity with practicality—managing to fuse innovative thoughts with insights from the market.
Identifying Market Needs
Understanding what the market craves is the first hurdle to cross. This involves comprehensive research, not just scraping the surface. Potential customers often have unsaid needs, which could be unearthed through surveys, direct interviews, or even analyzing online discussions. This is where the rubber meets the road; a keen sensitivity to current trends can set the stage for what shape your product will take. For instance, a fintech company might observe an uptick in users wanting seamless budgeting tools, indicating a gap in the current offerings. Retailers might notice that consumers are increasingly leaning towards personalized shopping experiences. By identifying these needs early, you can craft data products that truly resonate.
Formulating Data Products by Definition
Once market needs are identified, it is time to translate those insights into actual data products. This involves defining key features, scope, and problem-solving capabilities of your product. The goal here is to ensure that the product will not only fulfill user needs but will also stand out amid the clutter. A strong definition serves as a North Star, guiding development choices and ensuring everyone involved knows what they're working toward.
For example, if a company decides on a data analytics tool, it should clearly articulate whether it's addressing lagging sales, improving customer retention, or optimizing supply chain logistics. Ambiguity can result in a muddled product that fails to hit the mark, thus cutting down on the invested resources.
Gathering Stakeholder Input
Involving stakeholders during the ideation phase can illuminate paths often overlooked. Stakeholders can include employees, investors, or even end-users, and securing their input can be invaluable. Free-flowing dialogue encourages diverse perspectives, leading to well-rounded decision-making. Schema interviews or focus groups might reveal potential pitfalls or innovative concepts that could otherwise slip through the cracks.
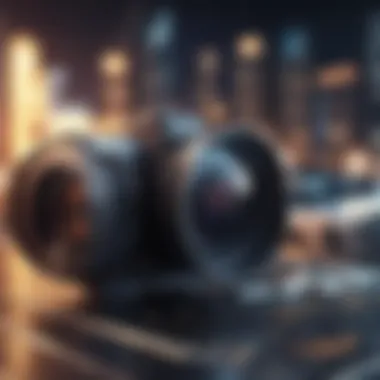
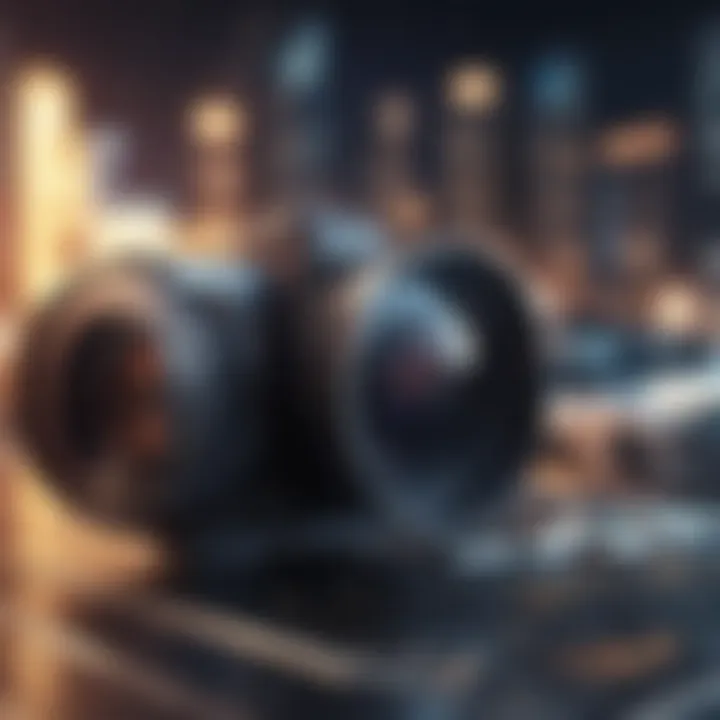
Here, the incorporation of Agile methodologies can be particularly beneficial. Engaging stakeholders regularly ensures the product remains relevant and meets expectations. By capturing their feedback, you not only refine your concept but also garner a sense of ownership among those involved, enhancing future collaboration.
"A product not based on user needs is merely an idea on paper."
In summary, Phase One of the Data Product Life Cycle highlights the significance of thoughtful conceptualization. By recognizing market needs, clearly defining the product, and involving stakeholders, you set a solid foundation for subsequent phases. This phase may feel like pulling teeth at times, but it has the potential to reveal the jewels hidden in consumer expectations. Understanding these elements can lead to a successful data product, ultimately driving better investment decisions and outcomes.
Phase Two: Development and Prototyping
The Development and Prototyping phase is a pivotal stage in the data product life cycle. It transforms abstract ideas from the previous phase into tangible products, setting the stage for their market introduction. This is where concepts begin to take shape and discerning whether a product can fulfill market needs becomes paramount. Without careful consideration during this phase, products may falter, information may be mishandled, and resources could go to waste. Let’s explore this in detail.
Selecting Appropriate Technology
In the realm of data product development, choosing the right technology is key. Various platforms and tools exist, each boasting unique features and capabilities. This selection process should not be taken lightly, as the technological framework selected can impact the product’s performance, scalability, and overall user experience.
Factors to consider include:
- Compatibility: Ensure the technology can seamlessly integrate with existing systems and data sources.
- Scalability: As the user base grows, the technology should adapt without crashing or slowing down.
- Support: Evaluate the community and documentation available for resolution of issues.
A common pitfall is overengineering. Sometimes, less complicated tools can perform just as well without the complexity. Hence, it’s wise to assess needs honestly before diving into advanced solutions. Start small, ensure it works, then evolve.
Creating a Minimum Viable Product (MVP)
The Minimum Viable Product (MVP) is often the backbone of product development in the data space. Aim to develop a product that has just enough features to satisfy early adopters and gather user feedback. This approach helps in validating assumptions about the market and understanding user preferences without committing too many resources upfront.
When creating an MVP, consider focusing on:
- Key Features: Identify core functionalities that solve specific user problems.
- Testing Hypotheses: Build to test assumptions and discover what resonates with users.
- Iterative Improvements: Use feedback from real users to guide the product’s features.
Launching an MVP signals a step toward real-world testing. It can expose unforeseen issues and lead to more informed iterations. The beauty of this method lies in its adaptability; it ensures you're not running too far down a path that may not be viable.
Iterative Testing and Feedback Mechanisms
Testing and feedback should be woven into the development tapestry. Instead of waiting until the end of the development cycle to gather user insights, integrating feedback throughout the process allows for more effective iteration.
Best practices include:
- User Testing Sessions: Engage potential users at various stages to gain insights.
- Surveys and Questionnaires: Use structured methods to gather specific data on user experience.
- Live Feedback Tools: Employ tools that allow you to identify issues in real-time during user interactions.
Ultimately, this approach ensures that the product is refined based on actual user needs rather than assumptions. Additionally, creating a culture that both values feedback and embraces change can foster a product that consistently meets market demands.
"In the game of product development, user feedback is your GPS; it guides you to your final destination through the twists and turns of market needs."
Phase Three: Launch and Market Prolusion
Launching a data product is akin to setting foot on a bustling marketplace with all eyes on you. It's a critical juncture, and it's where all the groundwork laid in the previous phases comes to fruition. At this point, the planning from ideation and the meticulous development all align into a coherent marketing strategy aimed to capture attention, convert interest, and ensure longevity in a competitive landscape.
Developing a Marketing Strategy
Crafting an effective marketing strategy is essential for the success of any data product. This strategy needs to resonate not just with potential users but also with stakeholders who might have vested interests.
- Target Audience Identification: Knowing your audience is half the battle. Identify demographics, preferences, behaviors, and pain points. Are you catering to investors looking for analytics tools? Perhaps brokers needing real-time data? Tailor your messaging accordingly.
- Unique Selling Proposition (USP): What makes your data product stand out? Define your USP clearly. This can include advanced features, superior data quality, or exclusive access. A well-articulated USP can pique interest effectively.
- Content Generation: Develop high-quality content to establish credibility. Blogs, whitepapers, and tutorials go a long way in educating potential users. High-ranking keywords in this content can also help boost online visibility, contributing positively to SEO metrics.
- Multi-Channel Approach: Leverage various platforms for your marketing efforts. Social media, email newsletters, webinars—each provides unique ways to engage with your audience and spread the word about your product.
Executing a Successful Launch
The actual launch event requires careful orchestration to ensure that everything from feedback to user engagement is up to par.
- Soft Launch vs. Hard Launch: Consider doing a soft launch, where a selected group of users can test the product and provide feedback before a larger release. This strategy can help iron out any wrinkles and address concerns early.
- Engagement Metrics: During the launch, pay attention to engagement metrics. Are users logging in? Are they providing feedback? Actively monitoring these metrics allows for swift adjustments in strategy to improve user experience.
"A successful launch is not just about the day; it's about ensuring that users feel heard and valued from the get-go."
- Promotion Efforts: As you launch, ensure there's sufficient promotion in alignment with your earlier marketing strategy. Share updates, milestones, and user testimonials across platforms to keep the momentum alive. Remember, word-of-mouth can be more powerful than one might think.
Initial User Acquisition and Retention Strategies
Acquiring users is the first hurdle, but keeping them around is where the real challenge lies. Retention strategies need to activate after the initial acquisition phase.
- Onboarding Experience: Create a seamless onboarding experience for users. A guided setup can help users navigate the product effectively, preventing them from feeling overwhelmed.
- Feedback Channels: Establish open channels for feedback—be it surveys, forums, or direct communication. It shows users their opinions matter, fostering loyalty.
- Regular Updates: Keep your user base engaged through regular updates about new features or improvements based on their feedback. This not only improves the product but also demonstrates to users that you are committed to continuous improvement.
- Community Building: Creating a community around your product encourages user interaction and shared experiences. This can be a forum, social media group, or even implementing user-generated content that celebrates the product’s use in real-world scenarios.
Each of these components is essential for the successful launch and market introduction of a data product. This phase is often where expectations meet reality; handled well, it can lead to growth and expansion, turning your data product into a trusted name in the market.
Phase Four: Growth and Scaling
In the data product life cycle, the phase of growth and scaling stands as a pivotal juncture. It's where a product begins to gain traction, moving from the hands of early adopters into the broader market. This is often a make-or-break time, as the choices made here could either catapult a product into success or let it flounder in obscurity. Understanding how to effectively analyze user feedback, leverage data for continuous improvements, and scale operationally are all fundamental aspects worth dissecting.
Analyzing User Behavior and Feedback
Gleaning insights from user behavior is essential in shaping a product's future. Every click, interaction, and stumbling block experienced by users offers a wealth of information that can guide modifications and enhancements. Tools like Google Analytics or Hotjar can provide a breakdown of how users interact with the product, allowing developers to spot trends and identify areas of friction.
- User Surveys: Gathering direct feedback through surveys can highlight user sentiments and expectations, helping guide development priorities.
- A/B Testing: Running tests on different features or layouts can yield real-time data on what resonates more with users, enabling a targeted approach to further improvements.
- Churn Analysis: Understanding why users exit or disengage helps in making strategic adjustments to retain users. Occasionally, it’s about refining the user experience rather than overhauling the entire system.
In this fast-paced environment, reacting promptly to user feedback and behaviors can distinguish a product from its competitors. Users are not just numbers; they are the lifeblood of a product, and acknowledging their experiences can lead to sustained growth.
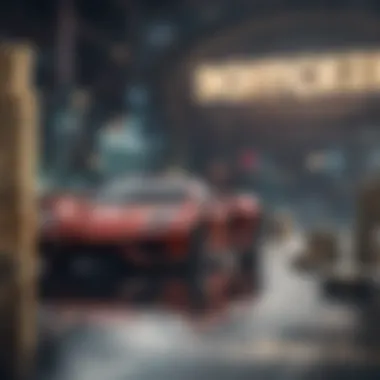
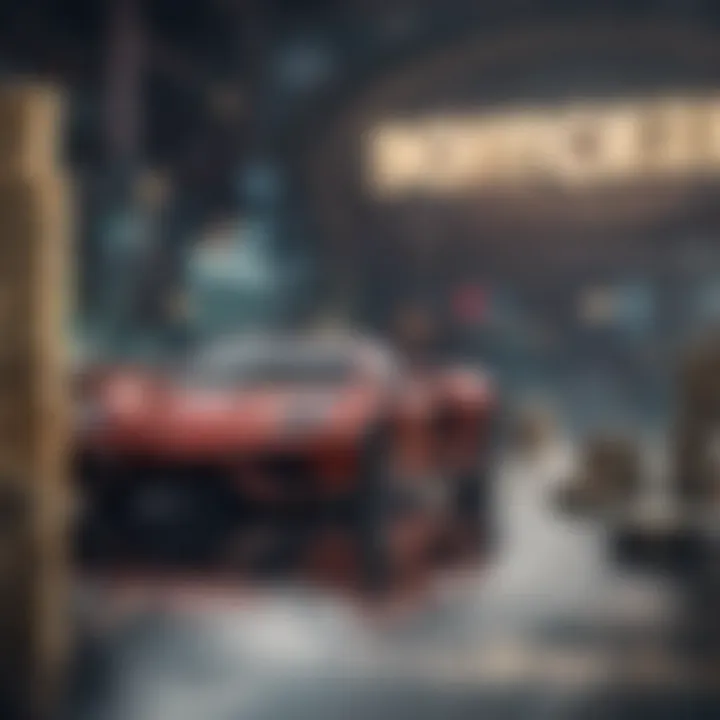
Leveraging Data for Continuous Improvement
The concept of continuous improvement revolves around the relentless pursuit of excellence. In the world of data products, this means harnessing the data generated by users to refine and enhance product offerings. Essentially, it’s about evolving the product in tandem with user needs and market trends.
- Data-Driven Decision Making: Utilizing analytics to inform decisions minimizes guesswork. By examining usage statistics and performance metrics, businesses can pinpoint which features add the most value.
- Feedback Loops: Establishing systematic feedback loops ensures that insights are gathered and implemented regularly, not just in isolated instances. Connecting user experiences to development teams maintains a user-centric product evolution.
- Benchmarking: Comparing performance with industry standards or competitors can shed light on opportunities for enhancement and innovation. This comparative approach can help push the product forward and identify gaps that can be filled.
The crux of leveraging data for continuous improvement lies in a proactive rather than reactive stance. Businesses that treat data as a strategic asset are often the ones who stay ahead of the curve.
Scaling Operations and Infrastructure
As growth accelerates, scaling operationally becomes critical. This involves not just expanding the user base but ensuring that the underlying infrastructure is robust enough to handle increased demand without falling apart at the seams.
- Cloud Solutions: Implementing cloud-based services can provide the necessary scalability while keeping costs manageable. This flexibility allows businesses to adjust resources based on real-time demand, without overcommitting.
- Automated Processes: Automating repetitive tasks can free human resources for more complex problem-solving, enhancing efficiency across the board. This could range from customer support chatbots to automated reporting systems.
- Partnerships: Aligning with partners who can provide complementary resources can facilitate smoother scaling. Whether through technology, distribution, or expertise, finding the right partnerships can amplify operational capacity.
Real growth often necessitates thoughtful scaling. Companies that invest in the right infrastructure today can avoid headaches in the future as customer bases expand and operations evolve.
A well-structured growth phase not only focuses on acquiring new users but also on enhancing the existing product based on user feedback and data while ensuring the operational backbone is solid.
In summary, phase four of the data product life cycle is not merely about riding the growth wave. It demands a nuanced understanding of user interaction, a commitment to continuous improvement through data, and a vigilant approach to scaling operations. Each subheading within this phase interlocks with the next, forming a cohesive strategy that ultimately positions a data product for lasting success.
Phase Five: Maturity and Optimization
In the data product life cycle, the Maturity and Optimization phase is akin to finding one's stride. By this time, a data product has often established its market presence, captured a loyal user base, and demonstrated its utility across various applications. Yet, just as a fine wine can always be improved, so too can a successful data product. This phase emphasizes the need to refine and enhance offerings, ensuring they not only meet current user demands but also adapt to the shifting landscape of technology and consumer expectations.
Evaluating Product Performance
Listening to the heartbeat of a product – that’s what evaluating performance is all about. Metrics play an essential role here; they not only indicate how well the product serves its users but also signal areas needing attention. Common evaluation methods include:
- User Engagement: Are users returning to the product regularly? Tracking daily active users (DAU) and monthly active users (MAU) can provide insight into engagement levels.
- Conversion Rates: For many products, understanding the journey from user interaction to desired actions is vital. Analyzing conversion rates can highlight where users drop off in the process.
- Customer Satisfaction: Gathering feedback through surveys or Net Promoter Scores can give a sense of user sentiment.
Implementing these metrics isn’t just about numbers; it’s about weaving them into the overall narrative of the product’s impact, aligning actions with documented outcomes, and making adjustments as needed.
Identifying Areas for Further Optimization
In the realm of optimization, standing still clearly won’t do. Unlocking further potential requires keen observation and a willingness to experiment. This might involve:
- Feature Enhancements: Sometimes, a simple tweak to an existing feature based on user feedback can significantly improve usability.
- Performance Improvements: Speed is of the essence. Whether it’s faster load times or reducing data processing delays, optimizing performance is crucial for user retention.
- Cost Efficiency: Reducing operational costs without sacrificing quality is a balancing act that can lead to profit maximization.Also, consider implementing A/B testing. By comparing two versions of a product, teams can pinpoint which changes resonate better with users, ensuring optimization efforts are data-driven rather than guesswork.
Maintaining Competitive Advantage
In the fast-evolving landscape of data products, maintaining a competitive edge is more than just keeping abreast of trends; it involves proactive strategy. Key strategies include:
- Regular Benchmarking: Compare against competitors, understanding their strengths and weaknesses, helps to identify gaps in one’s own offerings.
- Innovation Culture: Foster an environment where team members feel encouraged to propose new ideas or improvements, ensuring the product remains fresh in the marketplace.
- User-Centric Approach: Continual engagement with the user base can reveal insights into their changing needs and desires, which can drive the product road map.
"The secret to maintaining relevance lies not in the past successes, but in our ability to anticipate the future needs of our users."
In essence, the Maturity and Optimization phase is about evolving. It calls for a balance between reflection and innovation, grounding decisions in data while remaining agile enough to pivot in response to users' changing environments and preferences. The art of refining a data product during this vital phase can transform it from merely adequate to outstanding, ensuring its place in the market for years to come.
Phase Six: Decline and Sunset
Understanding the decline stage of the data product life cycle is crucial for anyone in the data-driven industry. When a product starts to lose its relevance or profitability, businesses face a pivotal choice: to sunset the old product or reinvigorate it. This phase is not merely a failure; instead, it holds valuable lessons for future projects. Effective handling of this decline ensures that resources are allocated wisely, avoiding unnecessary expenditure on products that no longer serve their purpose.
Recognizing Obsolescence Signals
Every data product isn't meant to last forever. Recognizing when it’s becoming obsolete can sometimes feel like finding a needle in a haystack. Some telltale signals indicate the product’s decline:
- Decreasing User Engagement: If user activity is waning, it’s a red flag. Look closely at statistics; if users aren’t interacting as much, something’s amiss. Perhaps the user needs are shifting, or other solutions have emerged in the marketplace.
- Customer Feedback: Pay attention to what your customers say. Trends in feedback, especially negative statements, can reveal underlying problems with the product or service.
- Market Trends: Keep an ear to the ground for changes in the industry. New technologies or methodologies can render your data product less competitive. Keep in mind what the competition is up to – they often hold the key to understanding your product's position.
Recognizing these signals promptly allows businesses to respond effectively rather than being caught flat-footed.
Strategizing for Transition
Transitioning from an old product presents its own set of complications. Here are some ways to strategize this delicate endeavor:
- Evaluate the Current Product: Take stock of resources. Is the data still valuable? Can it inform future projects, or is it better to lay it to rest? These questions guide decision-making the right way.
- Engage Stakeholders: This phase isn’t just about bottom lines; it’s also about the people involved. Communicating with clients, team members, and partners about the upcoming changes ensures a smoother transition. Their input could offer insights that may have been overlooked.
- Plan for Reallocation: When resources aren’t just going to evaporate, it’s important to allocate them wisely. Identify areas where the product’s data can contribute meaningfully, whether in innovative projects or refining other existing products.
Strategizing effectively shapes how the company emerges from the decline stage, positioning it better for future endeavors.
Phasing Out Procedures
After all the strategic planning, it's time to execute the phasing out. The steps involved in this process should be meticulously planned:
- Clear Communication: Users should always be informed about the winding down of a product well in advance. This transparency builds trust. Customers appreciate knowing what to expect and how they will be supported throughout the process.
- Transition Support: Offer customers alternative solutions or assistance in moving to other products. Whether it be through tutorials or dedicated support lines, ensuring a seamless transition can transform a difficult phase into an opportunity for customer loyalty.
- Data Migration: If applicable, ensure there’s a secure process for migrating data from the old product to newer ones or offering users data backup beforehand. This part is often overlooked, so addressing it can prevent chaos.
"A careful exit strategy can preserve relationships with customers long after the product is gone."
Engaging in these procedures with thoughtfulness can ultimately reflect positively on a company’s reputation and long-term strategy. In the end, recognizing decline doesn’t have to spell doom but can represent a chance for rejuvenation and strategic pivoting.
Challenges and Considerations in the Life Cycle
The data product life cycle is more complex than most people think. Within its many stages, one must grapple with numerous challenges and considerations that can make or break a project. Understanding these difficulties isn’t just about recognizing them; it's about knowing how to navigate them. Successful management of these challenges catapults the entire product through its life cycle, ultimately impacting its viability and acceptance in the marketplace.
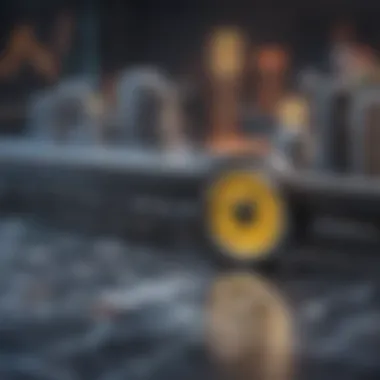
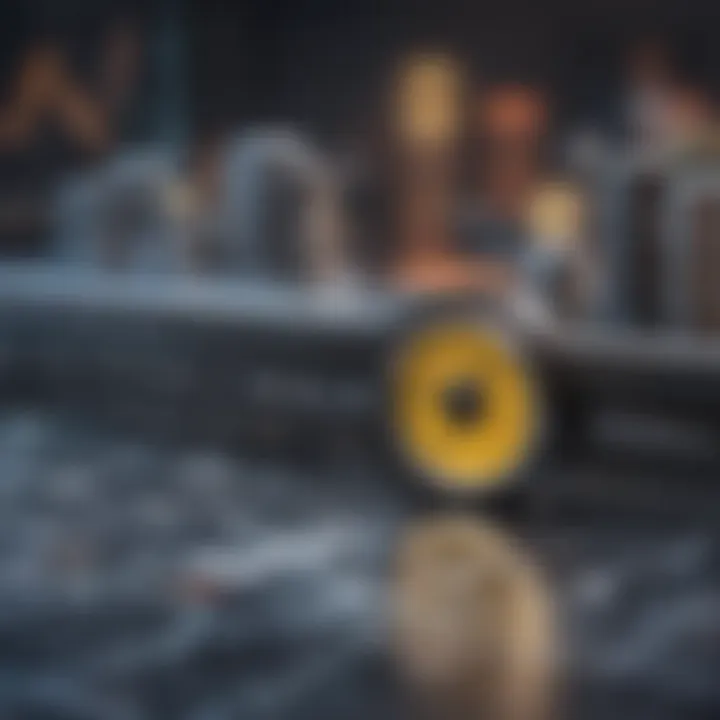
Data Quality and Governance Issues
Data quality is the bedrock of any data product. If the input data is unreliable or flawed, every decision based on that data becomes suspect. Poor data quality can lead to misinterpretations and misguided strategies, heavy financial losses, and, consequently, a tarnished reputation.
Consider the implications of inadequate governance. For instance, if your organization lacks clear data management protocols, it can result in data silos where information remains trapped and unusable. This situation not only stifles productivity but can also lead to significant compliance issues later on.
"Organizations must prioritize data governance and quality before rushing into product development. Cutting corners at this stage is akin to building a house on shaky ground."
Implementing established practices can include:
- Regular audits to assess data quality.
- Establishing a data stewardship team to oversee the governance framework.
- Utilizing data profiling techniques to enhance quality metrics.
Regulatory Compliance Challenges
In the digital age, navigating through the maze of regulatory frameworks can prove daunting. With GDPR in Europe and CCPA in California, data privacy laws are not just bureaucratic hurdles; they are essential frameworks that protect individuals.
Falling foul of these compliance regulations can lead to hefty fines and legal repercussions. Beyond the direct financial implications, non-compliance can also degrade customer trust and loyalty, which can be incredibly damaging in a competitive environment. When creating data products, organizations must understand the legal landscapes impacting operations.
A common approach to countering these challenges includes:
- Continuous monitoring of legal requirements for the jurisdictions involved.
- Engaging compliance officers early in the data product development phases.
- Conducting regular training for teams on data handling practices and compliance laws.
Addressing User Privacy Concerns
We live in an era where consumer awareness about privacy is at an all-time high. If organizations fail to address user privacy, they risk alienating their audience, resulting in lost revenues and heightened scrutiny.
Building product features with privacy in mind from the get-go can significantly enhance user trust. Utilizing transparency mechanisms—like clear privacy policies and accessible opt-in/opt-out choices—allows users to feel more in control of their data.
To ensure privacy is a priority, teams can:
- Implement privacy-by-design principles during the development phase.
- Conduct privacy impact assessments to gauge how data will be used.
- Foster a culture of respect for user data across all departments.
Addressing these challenges is paramount along the data product life cycle. Being proactive about data quality, regulatory compliance, and user privacy enables organizations to develop innovative solutions while maintaining the trust and loyalty of their consumers. Keeping these elements in focus ultimately leads to a smoother and more successful product journey.
Future Trends in Data Product Life Cycle Management
The landscape of data product development is constantly shifting, impacted by technological advancements, evolving user needs, and regulatory changes. Understanding the future trends in data product life cycle management is crucial for anyone involved in leveraging data for decision-making and strategic advantage. As we navigate through this era of rapid change, several key trends emerge that not only shape the direction of data products but also offer opportunities for enhanced performance and growth.
Impact of Artificial Intelligence
Artificial Intelligence, or AI as it’s often codified, has become a cornerstone in many industries, including data product management. Its role in automating processes, enhancing user experiences, and providing actionable insights is unmatched. For instance, consider the realm of customer service powered by AI chatbots, which revolutionize interaction by providing instant feedback and data-driven solutions.
AI facilitates better data analytics. By employing machine learning algorithms, companies can predict trends, identify anomalies, and fine-tune their products based on user interaction. This data-driven decision-making ensures that the offerings evolve in response to real-time feedback, thus enhancing relevance in the market.
To illustrate, think of how Netflix recommends shows based on individual viewing patterns. This not only keeps users engaged but also improves subscriber retention. Companies that harness AI effectively are likely to create products that resonate more profoundly with their target audience, fostering loyalty and sustained interest.
Potential for Automation in Management
Automation stands as another game changer in data product life cycle management. By streamlining tasks, automation reduces the manual effort required at various stages—from development to monitoring product performance. This is particularly relevant in handling data quality checks, deployment, and updates.
For example, automated testing frameworks can ensure consistent quality of product releases while minimizing human error. Imagine a financial advisor using a tool that can automatically generate reports on data trends, saving hours of manual work and allowing for focus on strategic client interactions.
There's also the part about integrating Continuous Integration/Continuous Deployment (CI/CD) pipelines, which allows teams to deploy updates faster while maintaining the integrity of the product. Automation is not merely a trend; it’s becoming a necessity for efficiency and precision.
Emphasis on Ethical Considerations
As data product life cycles evolve, ethical considerations are gaining traction. Stakeholder awareness regarding data privacy and security is ballooning, and with regulations like the GDPR looming, it’s imperative for data managers to prioritize ethics in their strategies.
Ethical data sourcing, consent, and transparency are no longer optional. Companies must ensure that they are not only compliant with laws but also respecting user preferences and creating trust. This isn’t just about avoiding legal problems; it’s about building a reputable brand that users can rely on. For instance, Facebook’s data sharing scandal brought a wave of scrutiny, showcasing the perils of neglecting ethical standards.
To this end, companies are encouraged to implement frameworks that prioritize ethical engagement with both data sources and users. Transparency in how data is collected and used can significantly bolster consumer trust, leading to better user engagement and loyalty.
In summary, the future of data product life cycle management hinges on integrating AI, automation, and ethical practices into everyday operations. These elements not only address current challenges but also pose great potential for transformative growth in the data landscape.
Concluding Thoughts on the Data Product Life Cycle
Navigating the intricacies of the data product life cycle is akin to sailing in uncharted waters; it requires not only robust knowledge but also the agility to adapt to changing tides. The final phase of the cycle serves as a reflective lens that brings clarity to the journey from inception to maturity and beyond.
Revisiting the Importance of Each Phase
Each phase throughout the data product life cycle holds critical importance, acting as building blocks that construct the foundation of a successful product. From ideation where ideas are born, to the final phase of decline where strategies are reevaluated, all steps play a role in shaping the overall trajectory of the product.
- Awareness of Market Needs: Understanding the market at the outset means that the rest of the cycle is informed by real demands. Jumping straight to development, without this knowledge, can lead to missteps.
- Effective Prototyping: The transitions from concept to a fully realized product depend heavily on how well the prototype is received and improved upon. Iteration is inevitable, and these tweaks can mean the difference between success and failure.
- Launch and Growth: A well-executed launch often sets the momentum for growth. Initial feedback helps refine product features and user engagement strategies, keeping the product relevant.
- Decline Management: Recognizing when a product is nearing its twilight ensures that resources can be reallocated to foster new innovations rather than dragging a declining product.
"The life cycle is not merely a process; it’s a monument to adaptability and foresight. Every phase we step through informs the next."
Each element interconnects, emphasizing the need for a holistic understanding of the life cycle. Investors and analysts must pay keen attention not just to one phase but to the overarching journey that a product takes, allowing for insightful forecasting and strategic planning.
Encouraging Continuous Learning and Adaptation
In an era where data environments fluctuate unpredictably, continuous learning becomes vital for anyone involved in the data product life cycle. The market’s pulse shifts swiftly; thus, staying informed is not just beneficial—it’s crucial.
- Emphasizing Adaptability: Successful data products evolve. Stakeholders need to remain flexible, employing a mindset that values innovation and embraces change, viewing challenges as opportunities for growth.
- Incorporating Feedback Loops: Regularly collecting and acting on user feedback can unveil insights that spark significant improvements. This practice promotes a culture of responsiveness and reinforces user trust.
- Upholding Ethical Standards: Maintaining a commitment to ethical considerations and data privacy resonates with users. As they become more aware of their rights and data usage, companies must adapt accordingly, ensuring compliance with regulations.
For stakeholders, the challenge lies in fostering a culture of learning, where the lessons extracted from each phase are woven into the fabric of future endeavors. Fostering continuous adaptation will allow organizations to thrive in the ever-evolving landscape of data products.
Ultimately, the data product life cycle’s conclusion is not the end, but a segue into new opportunities. Failure to acknowledge this can lead to stagnation. By keeping an open mind and staying engaged with trends and user needs, investors and teams can pursue continuous improvement, ensuring that they remain at the forefront of their industry.