Impact of Data Analytics on Finance Industry


Intro
In the fast-paced world of finance, data analytics plays a critical role that cannot be overstated. Financial institutions across the globe are delving into the vast ocean of data they generate and collect, aiming to pull out actionable insights that can guide decision-making, risk assessment, and customer engagement. The application of data analytics is reshaping the landscape of finance, acting as an essential tool for investors, brokers, and financial advisors. This powerful technology is not just about numbers; it’s about navigating complex financial waters with clarity and precision.
Given the increasing abundance of data available today, understanding how to leverage it effectively is vital. In this exploration, we will dissect how data analytics is transforming the finance industry, highlighting key trends, practical applications, and the ethical considerations that accompany this endeavor. Once viewed as a mere support function, data analytics has emerged as a cornerstone of strategic initiative in financial practices, providing a lens through which investors can assess opportunities and risks.
Let’s take this journey step by step, diving into the nitty-gritty of how data-driven insights are bridging the gap between raw information and sound investment decision-making.
Investment Dictionaries
Key Terms and Definitions
Understanding the jargon around data analytics and finance is crucial. Below are some key terms defined clearly to assist in navigating this realm:
- Data Analytics: The science of examining raw data to draw conclusions about that information.
- Predictive Analytics: A form of data analytics that focuses on forecasting future outcomes based on historical data.
- Risk Management: The process of identification, assessment, and prioritization of risks followed by coordinated efforts to minimize or control the probability of unfortunate events.
- Big Data: Extremely large datasets that may be analyzed computationally to reveal patterns, trends, and associations.
The banking sector, for example, employs these analytics to mitigate fraud and analyze customer behavior, thereby tailoring services to meet individual needs. On the other hand, investment firms use predictive analytics for market predictions, which helps in forming more accurate financial models.
Practical Applications of Investment Terms
To understand how these elements intertwine within financial practice, consider the following applications:
- Fraud Detection: Financial institutions utilize data analytics to identify potentially fraudulent transactions in real-time, significantly reducing losses.
- Customer Segmentation: By examining data patterns, firms can segment their customers to tailor marketing strategies.
- Portfolio Management: Data analytics provides investment managers with insights into market trends, enabling them to optimize portfolio performance.
- Credit Scoring: Banks analyze customer data to determine creditworthiness more accurately, thus minimizing risk.
Engaging with these applications demystifies the role of data and its relevance to intelligent investment.
Expert Advice
Strategic Insights for Different Investment Types
When considering how best to employ data analytics, it’s essential for investors to align their strategies with the type of investment they pursue. For example, in real estate, analytics can optimize property purchases based on market trends and demographic insights. In stocks, recognizing volatility patterns through analytics can help investors make timely decisions.
- Diversify understanding across various investment vehicles to utilize analytics effectively.
- Regularly update data sources and analytics tools to reflect the current market conditions.
Tips for Evaluating Financial Products
- Compare Historical Data: Look for data that shows the consistency of performance.
- Consider Risk Levels: Analyze how products have responded to market fluctuations in the past.
- Review Customer Feedback: Insights from other users can guide decision-making in choosing financial products.
In summation, the interplay of data analytics in finance is not just a trend; it’s fundamentally changing the way financial institutions operate and how investors navigate their portfolios. As this field continues to evolve, staying informed and adaptable can make all the difference.
"Data analytics isn’t just about crunching numbers; it’s about harnessing the pulse of the market to make informed decisions."
In the subsequent sections, we will continue to uncover emerging trends and the ethical landscape surrounding data analytics in finance.
Understanding Data Analytics in Finance
Understanding data analytics in finance isn't just a buzzword tossed around in boardrooms—it's a vital cornerstone for modern financial institutions looking to thrive in a cutthroat industry. This section will unravel what data analytics truly means within finance, along with its historical evolution and significance to today's market dynamics.
Definition of Data Analytics
Data analytics, in its simplest form, refers to the systematic computational analysis of data. In the finance sector, it involves scrutinizing various data sets, determining patterns, trends and gathering insights that help institutions make informed business decisions. Whether it’s evaluating investment opportunities or optimizing operational efficiency, data analytics serves as the compass steering financial strategies.
The primary goal here is to extract actionable intelligence from what might seem like a maze of numbers and figures. For example, when banks assess potential loan applications, they dive deep into applicants' financial histories, using analytics to forecast repayment behaviors. By leveraging tools that analyze credit scores, income histories, and even social media behavior, they can better predict risk and reduce default rates.
Historical Context of Analytics in Finance
To appreciate the contemporary role of data analytics, one must delve into its roots. In the earlier days of finance, decisions were often based on gut feelings or historical precedents. The introduction of computers revolutionized this thinking.
Back in the 1970s, when banks started adopting rudimentary computer systems, they gained the ability to process larger data sets more efficiently. Fast forward a couple of decades, and the emergence of software solutions like spreadsheets began leading finance professionals to analyze data more deeply. Nowadays, it's hard to envision the financial landscape without sophisticated algorithms and programs.
In the 1990s, with the dawn of the internet, online trading platforms began collecting massive amounts of transaction data. This surge spurred an era of real-time analysis, enabling traders to react swiftly to market changes. The transformation didn’t stop there; with the tech boom of the early 2000s, data analytics evolved to include advanced statistical methods, allowing for increasingly precise forecasting and risk assessment models.
A quote that encapsulates this transition aptly states, > "Finance is no longer about just numbers—it's about understanding what those numbers really mean."
As we move into an era dominated by big data, financial institutions find themselves at a pivotal point. The maturation of analytics technology has opened doors to greater efficiencies and impressive insights, steering the financial sector toward a future that's heavily data-driven. Thus, understanding this evolution is crucial for investors and financial professionals keen on staying ahead of the curve.
Key Applications of Data Analytics


Data analytics has profoundly changed the landscape of the finance industry. It's not just about numbers and spreadsheets anymore. Financial institutions are tapping into analytics to tailor their services, minimize risks, and improve strategic decision-making. This section will cover the major applications of data analytics within finance, emphasizing their roles in risk management, decision-making enhancement, and customer insights.
Risk Management Strategies
Credit Risk Analysis
Credit risk analysis is a cornerstone of financial stability. It focuses on determining the likelihood that a borrower may default on a loan or credit obligation. For financial institutions, understanding this aspect is vital. They can avoid potential losses by assessing the creditworthiness of their clients.
A key characteristic of credit risk analysis is its reliance on qualitative and quantitative data. A lender might analyze credit scores, income levels, and even transaction history to gauge risk. This multifaceted approach distinguishes it as a popular selection for financial operations. One unique feature is the use of predictive modeling, whereby past data informs future expectations. However, data quality is crucial — any gaps can lead to flawed assessments, which could be a significant disadvantage.
Market Risk Assessment
Market risk assessment deals with the effects of external factors on the financial markets. This includes shifts in interest rates, currency fluctuations, or even sudden stock market declines. It’s instrumental because it helps institutions make strategic decisions by identifying potential market vulnerabilities.
The primary trait of market risk assessment is its comprehensive nature. It not only considers internal data but also external macroeconomic indicators. This characteristic makes it a potent tool for a broader understanding of market dynamics. A notable feature here is scenario analysis. Financial entities employ simulations to gauge potential impacts of adverse conditions. That said, it can sometimes lead to over-reliance on models, which may not capture real-world unpredictability.
Enhanced Decision Making
Investment Strategy Optimization
Investment strategy optimization uses data analytics to evaluate and improve investment opportunities. By examining trends, past performances, and forecasting potential outcomes, investors can maximize their portfolio returns.
The key essence of this approach lies in its systematic nature. Rather than simply guessing or following intuition, analytic methods lead to informed decisions. One unique aspect is the ability to adjust strategies in real-time based on fresh data. This flexibility can be immensely advantageous, particularly in volatile markets. On the downside, heavy reliance on algorithms can be risky if market conditions shift abruptly, causing systems to lag.
Portfolio Management
Portfolio management encompasses the art and science of making investment decisions. Data analytics aids portfolio managers in diversifying assets, choosing optimal combinations, and balancing risk versus return. Its contribution here is significant, as it brings rigorous analysis to traditionally subjective choices.
A critical characteristic of effective portfolio management is its adaptability. Through continuous data analysis, financial experts can shift their strategies sooner rather than later. A captivating feature is performance attribution, which sheds light on how distinct assets contribute to overall results. However, the trade-off often lies in the potential for information overload — too many data points can lead to decision paralysis or second-guessing.
Customer Insights and Engagement
Behavioral Analytics
Behavioral analytics dives into understanding customer actions and tendencies. In finance, understanding how clients behave can unlock avenues for improved service offerings. When financial institutions recognize customer preferences, they can tailor their products to meet those needs.
A prominent characteristic of behavioral analytics is its focus on real-time data. By analyzing transactions as they occur, organizations can engage customers with relevant offers. This immediacy is particularly beneficial for maintaining customer loyalty. However, a caveat is that extensive tracking might raise privacy concerns if not handled with care.
Customer Segmentation
Customer segmentation is about dividing clients into distinct groups based on shared characteristics, such as behaviors or financial needs. This process allows institutions to target specific demographics more effectively.
A key strength of customer segmentation is its precision. By pinpointing the specific needs of various groups, financial institutions can provide personalized services. An appealing feature of this approach is its scalability — strategies can be adjusted as market conditions or customer behaviors evolve. Nonetheless, poorly executed segmentation can result in alienating clients or missing valuable opportunities, marking it as a potential drawback.
In summary, the applications of data analytics in finance are vast. From managing risks to fortifying decision-making and enhancing customer interactions, analytics plays a pivotal role. It allows financial institutions not just to survive but to thrive in an increasingly competitive landscape.
Technological Advancements in Data Analytics
The landscape of finance is shifting at an unprecedented pace, largely buoyed by technological advancements in data analytics. Understanding how these developments shape the finance industry is crucial. Modern finance relies on data to inform decisions. The ability to process and interpret vast amounts of data has revolutionized traditional practices. With every tick of the stock market, data analytics plays a pivotal role in driving efficiency and precision. This section examines significant technologies making waves in this arena.
The Role of Artificial Intelligence
Artificial Intelligence (AI) has emerged as a cornerstone in the world of data analytics, particularly within finance. Its influence cuts across numerous functions, ushering in a new era where machines become increasingly adept at analyzing data patterns that would take humans weeks or even longer to process. AI algorithms sift through mountains of structured and unstructured data. By learning from past behaviors, it aids in predicting market trends and economic shifts.
For instance, AI-driven models can enhance fraud detection by identifying outlier transactions that could indicate suspicious activities. These systems evolve continuously, learning from new data and improving their accuracy over time.
"AI is not only about automation; it's about enabling informed decision-making at lightning speed."
Machine Learning in Financial Forecasting
Machine Learning (ML), a subset of AI, has transformed financial forecasting. Unlike traditional forecasting methods based merely on historical data analysis, ML algorithms delve deeper. They can uncover hidden correlations and patterns that were previously unseen. By analyzing real-time market data, such as trading volumes and price fluctuations, machine learning models produce forecasts that adapt to ongoing market changes.
Furthermore, such models can automate the search for optimal investment strategies, allowing financial professionals to allocate resources more effectively. The predictive nature of machine learning ensures that investors can respond quickly to market dynamics, giving them a competitive edge.
- Benefits of using Machine Learning:
- Enhanced accuracy in risk assessment
- Continuous refinement of predictive models
- Automation of data analysis tasks
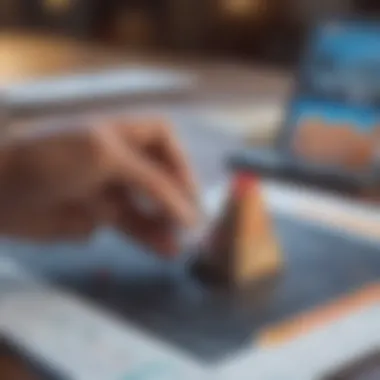
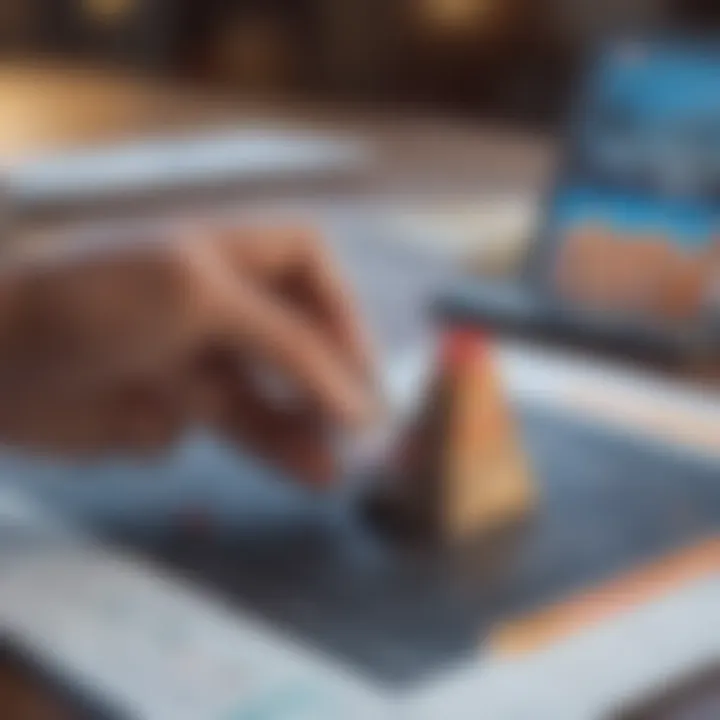
Big Data Analytics and Its Impact
Big Data analytics represents another significant leap forward. The rise of data generation—think social media interactions, online transactions, and IoT devices—provides financial institutions with unprecedented amounts of information. Harnessing this information allows firms to glean insights into customer behaviors, market trends, and overall economic conditions.
For instance, companies such as Amazon use big data analytics to personalize recommendations based on individual user preferences. The finance industry follows suit, leveraging similar techniques for improved customer experience and retention. By utilizing big data, institutions can not only better understand customer needs but also anticipate future behaviors based on past interactions.
Moreover, Big Data aids in improving operational efficiency. With instantaneous data processing, firms can make quick and informed decisions, ultimately leading to higher profitability. This technology not only enriches the financial landscape but also introduces challenges regarding data management and security that need to be addressed.
- Key aspects of Big Data in finance:
- Real-time analysis for better decision making
- Enhanced customer engagement through targeted marketing
- Risk mitigation through sophisticated analytics
In summary, the rapid advancements in data analytics technologies, particularly AI, Machine Learning, and Big Data, are reshaping the finance sector. These tools are indispensable for finance professionals seeking to navigate the complexities of the current market landscape. Investors and financial analysts must embrace these technologies to not only stay ahead but also thrive in an evolving environment.
Trends in Data Analytics for Finance
In today's fast-paced financial markets, staying ahead of the curve is not merely desirable; it’s essential. The integration of data analytics is transforming how financial entities operate, providing them with the tools to make informed decisions amidst a whirlwind of market data. By recognizing and adapting to emerging trends in data analytics, firms can improve their services and directly influence their bottom line. Let’s explore some of the key trends that are shaping the future of finance, with a specific focus on how organizations can leverage these advancements.
Cloud Computing and Data Storage Solutions
The rise of cloud computing has revolutionized the storage and management of large datasets in the finance sector. By shifting to cloud-based solutions, financial institutions achieve vast improvements in scalability and flexibility. This means they can process and analyze gigantic volumes of data without the limitations imposed by traditional on-premises servers.
Moreover, cloud storage enables organizations to engage in collaborative data analytics across geographically dispersed teams. As folks like to say, "A problem shared is a problem halved." By leveraging such technology, analysts across various departments can work together seamlessly, sharing insights in real-time.
Here are some key benefits of cloud computing in finance:
- Cost efficiency: Reduces the need for extensive in-house IT infrastructure.
- Enhanced security: Many cloud providers offer advanced security measures that can often surpass those of individual firms.
- Data accessibility: Facilitates access to real-time data, enabling quicker decision-making.
Real-Time Data Processing
In the financial world, timing is everything. Real-time data processing allows businesses to analyze data as it comes in rather than waiting for batch processes to finish. This trend is crucial for risk mitigation, fraud detection, and market evaluation. Instant access to live data allows financial advisors and traders to make timely decisions that could mean the difference between profit and loss.
Moreover, firms can utilize streaming analytics to identify trends and anomalies as they happen. This means that businesses can achieve a more proactive approach to data management. Think of it as having a front-row seat to the show, where every change can be addressed promptly.
Predictive Analytics for Future Investment Trends
Predictive analytics isn’t just a buzzword; it’s the backbone of strategic investment planning. By leveraging historical data and advanced mathematical models, financial institutions can anticipate future market movements and identify investment opportunities before they occur. Essentially, predictive analytics serves as a crystal ball for investors.
Utilizing machine learning algorithms helps in optimizing investment strategies by analyzing vast amounts of data and recognizing patterns that might be invisible to the human eye. This ability to predict market trends helps in:
- Assessing potential risks associated with various investments.
- Tailoring investment strategies to specific customer profiles.
- Crafting personalized financial advice that aligns with investor goals.
"If you want to predict the future, create it." This aptly describes the way forward for investors in navigating uncertainty using advanced data solutions.
The ongoing evolution of these trends in data analytics for the finance sector is not just an incremental shift; it’s a paradigm shift that empowers financial institutions to operate more efficiently and responsively. By embracing these trends now, firms position themselves for success in the future.
Challenges and Limitations
In the pursuit of leveraging data analytics for enhanced decision-making in finance, several challenges and limitations emerge that financial institutions must navigate carefully. These hurdles are not just mere obstacles; they speak volumes about the intricate balancing act between harnessing data's potential and adhering to responsible practices. This section shines a light on the prominent data privacy concerns, the integration of legacy systems, and the skill gaps in data analytics. Understanding these issues is vital for investors, financial advisors, brokers, and analysts aiming to maximize the benefits of data analytics in a prudent manner.
Data Privacy Concerns
Data privacy is akin to walking a tightrope for financial institutions. On one hand, vast troves of personal and sensitive information drive insight and growth. On the other hand, mishandling such data can lead to catastrophic breaches of trust and hefty regulatory fines. The stakes are high. Institutions must navigate legislation like the General Data Protection Regulation (GDPR) and California Consumer Privacy Act (CCPA), which underscore the necessity for enhanced safeguards.
The crux of the matter lies in balancing data-driven strategies with ethical stewardship. Here are a few considerations:
- Informed Consent: Customers should have a clear understanding of how their data will be used, reinforcing trust.
- Data Minimization: Collecting only what's necessary minimizes risks related to sensitive information.
- Transparency: Institutions must operate openly regarding their data policies, thus bolstering credibility.
If financial institutions neglect these concerns, they risk not just their reputation but also their bottom line. The risks associated with data breaches far outweigh potential gains from unregulated data use.
Integration of Legacy Systems
Another significant hurdle is the integration of legacy systems. Many financial institutions still rely on outdated infrastructure, which often lacks compatibility with modern data analytics tools. This creates a perfect storm of inefficiency and risk. Integrating new technologies into these aging systems is a monumental task that becomes an albatross around the neck of progress.
Considerations regarding legacy systems include:
- Costly Voyages: Upgrading or replacing underperforming systems can drain financial resources, diverting funds from innovation.
- Operational Disruption: Implementing changes can lead to temporary slowdowns, shifting the attention away from core business goals.
- Skill Requirements: Employees may need retraining to adapt to new systems, stretching the already strained resource pool.
Skill Gaps in Data Analytics
Finally, the skill gaps in data analytics present another formidable challenge. The finance industry is in dire need of professionals skilled in interpreting data and extracting actionable insights. Too often, firms grapple with a shortage of qualified analysts who can marry financial knowledge with data science expertise. This competency gap is a double-edged sword. Key points to consider:
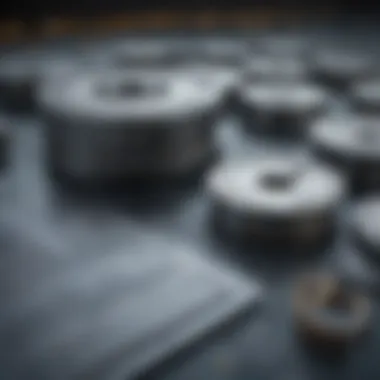
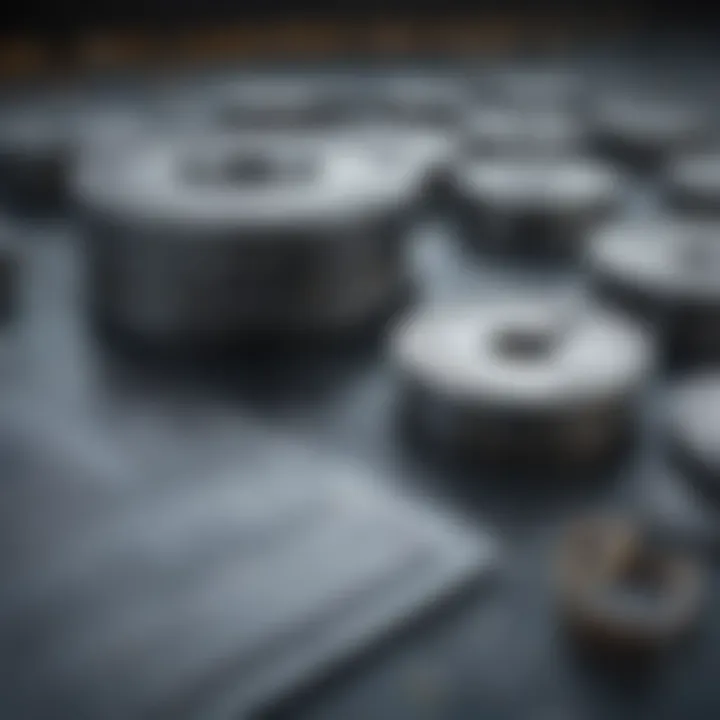
- Talent Acquisition: Companies face fierce competition for data talent, resulting in inflated salaries that can bust budgets.
- Continuous Learning: With technology evolving at breakneck speed, ongoing education is paramount. Employees need regular training to keep pace with the rapidly changing landscape.
- Interdisciplinary Approach: Integrating knowledge from finance, technology, and statistics is essential for unlocking the full potential of data analytics.
The challenges presented in this section are interlinked and exacerbate each other. Navigating these limitations while striving for progress is no small feat. However, acknowledging and addressing them head-on is crucial for any financial institution looking to thrive in the world of data-driven finance.
Ethical Considerations in Data Analytics
In today's increasingly data-driven financial landscape, ethical considerations stand out as critical cornerstones shaping the direction of analytics. The implementation of data analytics within finance offers enormous potential for improved decision-making, risk mitigation, and personalized customer experiences. However, the responsibilities tied to ethical data usage cannot be brushed aside. It is vital for financial institutions to navigate the complex waters of data ethics, as the stakes are high—not just for profitability, but for public trust and compliance with regulations.
Ethical Data Usage
The notion of ethical data usage extends beyond mere compliance with laws. It's about fostering a culture where customer data is treated with respect and integrity. Financial firms tap into vast pools of data every day. They analyze spending patterns, investment behaviors, and even personal demographics. In the quest to harness insights, companies often stand at the crossroads of functionality and ethics.
Here are some key aspects that highlight the importance of ethical data usage:
- Consent: Always obtain informed consent before collecting personal data. It’s more than a bureaucratic checkbox; it’s about ensuring clients are on board with how their data will be used.
- Purpose Limitation: Ensure that data collected serves a specified, legitimate goal. Unfettered access to data could lead to misuse with potentially harmful implications for customers.
- Data Minimization: Only collect data that is necessary for the task at hand. Avoid the temptation to gather data en masse, as this could lead to ethical pitfalls.
- Data Anonymization: Where possible, anonymize data to guard personal identifiers. This practice protects clients while still providing valuable insights.
Adherence to these principles not only bolsters a firm’s reputation but also fosters customer loyalty, creating a win-win scenario.
Transparency and Accountability
Transparency and accountability complement ethical data usage like two peas in a pod. Customers today are more data-savvy and expect honest communication regarding how their data is used. This expectation creates both challenges and opportunities for financial services.
- Open Communication: Financial institutions should maintain open channels regarding their data practices. Whether explaining algorithms that influence investment outcomes or detailing risks associated with data usage, clarity is key.
- Policy and Governance: Establishing robust data governance frameworks ensures that everyone, from entry-level analysts to C-suite executives, understands their role in ethical data handling. This is crucial in preventing incidents of misuse or data breaches.
- Regulatory Compliance: It’s impossible to overstate the worth of staying on the right side of regulations like GDPR or CCPA. Complying with these frameworks serves as a benchmark of responsibility.
- Accountability Mechanisms: Implement measures to hold teams accountable for ethical data practices. This might involve regular audits, feedback loops, and clear repercussions for unethical behavior.
At the end of the day, integrity in data usage works both ways—financial institutions owe it to their customers to act responsibly, and clients should hold firms to that standard.
The Future of Data Analytics in Finance
As the landscape of finance continues to evolve with technology, the future of data analytics looks promising and pivotal. Financial institutions are not only relying on past data but also looking forward to how real-time insights can reshape their strategies. The importance of understanding this topic cannot be overstated—it’s about preparing for a world where adaptability and insight-driven decision making are the names of the game. Financial analysts and investors alike have already begun to reap the benefits of innovative analytics methodologies, which emphasize agility and precision in operations.
Predictions and Upcoming Innovations
The potential for innovation in data analytics is vast, with several predictions indicating significant changes in how data will be utilized in this sector. One significant area is the integration of blockchain technology. It promises to offer transparency and security of data transactions, leading to trustworthy analytics. With blockchain, financial institutions can process transactions swiftly while ensuring integrity—an important aspect when analytics impacts financial decisions.
Another critical innovation lies in the realm of quantum computing. This technology could process immense datasets at unprecedented speeds, allowing for more complex and timely predictive models. Imagine being able to analyze decades of market data in a matter of minutes. This capability would enable firms to respond to market shifts swiftly, increasing their competitive edge.
Moreover, firms are likely to invest heavily in automated analytics tools. These mechanisms can help detect anomalies and provide insights without substantial human intervention. As a result, many mundane tasks could be streamlined, enabling analysts to focus on deeper strategic analysis.
"The swift evolution of data analytics shuffles the chessboard; it’s up to the players to capitalize on the new moves."
Despite the optimism, industries must approach these innovations with caution, particularly regarding ethical considerations. Ensuring responsible use of data remains paramount as new technologies come into play.
Resilience in Market Volatility
Market volatility is an inescapable reality for financial institutions. However, data analytics equips these organizations with the tools to build resilience against fluctuations. By leveraging predictive analytics, firms can anticipate downturns and react timely, minimizing potential losses. These anticipative measures allow businesses to navigate through uncertainty—an invaluable asset in today’s economic climate.
Some analysts suggest that the greater integration of real-time data systems will enable immediate responses to market changes. When institutions can act on current data more than ever before, they can not only mitigate risks but also identify new opportunities in real time.
Furthermore, the adoption of scenario analysis tools significantly aids in understanding various market behaviors. These strategies allow institutions to model potential outcomes and assess how certain variables might influence their investments.
To wrap it up, the future of data analytics in finance hinges on innovation, adaptability, and ethical responsibility. As this field continues to blossom, insights gained will undoubtedly shape investment strategies and operational processes, ensuring that those who stay ahead of the curve will thrive in the competitive landscape.
End
In today's finance landscape, data analytics stands as a pivotal pillar, underpinning numerous processes that drive the industry forward. The narrative we have woven throughout this article highlights various dimensions of data analytics, from risk management strategies to enhanced decision making and customer insights. Investing time and resources into these data-driven approaches isn’t merely an option anymore; it’s a necessity for financial institutions aiming to stay competitive.
Importance of Data Analytics
The significance of data analytics in finance cannot be overstated. It empowers firms to make informed decisions based on hard evidence rather than gut feelings. By examining patterns and trends, institutions can predict market behaviors and adjust their strategies accordingly. This, in turn, leads to better risk management, where firms can mitigate potential losses before they arise.
Financial analysts and advisors are gaining an edge as they harness algorithms and models to interpret massive datasets with unprecedented speed. This shift toward data-centric operations enables them to provide tailored recommendations for investors, adapting to their specific financial situations and goals.
Key Benefits
- Enhanced Decision Making: With real-time data at their fingertips, decision-makers can respond promptly to market changes, ensuring that investment strategies are aligned with current conditions.
- Improved Customer Experience: Tailored services that stem from behavioral analytics help understand clients better, fostering greater trust and engagement.
- Risk Mitigation: Utilizing predictive analytics allows organizations to foresee potential downturns in the market, helping safeguard assets and increasing resilience.
Considerations Moving Forward
As we look ahead, understanding the ethical implications of data usage becomes paramount. Balancing the benefits of data analytics with the risks of privacy breaches is a tightrope walk that financial institutions must navigate carefully. Transparency in how data is acquired and utilized will bolster customer trust, pivotal for establishing long-term relationships in finance.
Financial institutions must prioritize building a culture of compliance regarding data policies, ensuring that all operations align with applicable regulations. A focus on continuous education in data skills among employees will also be essential for keeping pace with ongoing developments in technology.
"Analytics is not just about the data; it’s about turning that data into actionable insights that guide strategic decisions."