Calculating Average: A Comprehensive Guide
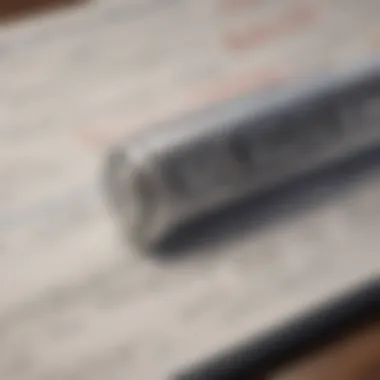
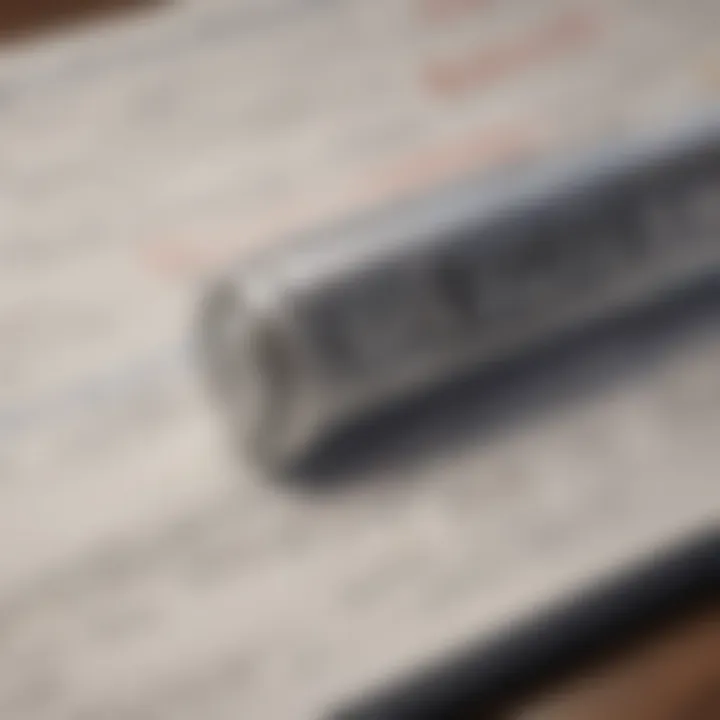
Intro
Calculating averages is a cornerstone of understanding data, whether in daily observations or financial decision-making. The term average often evokes thoughts of simple mathematics, but it plays a manifold role in various contexts. From analyzing daily expenses to evaluating investment portfolios, averages help to provide clarity and insights where data can seem overwhelming. This guide aims to break down the intricate layers of the averageâfocusing on mean, median, and modeâilluminating their significance, methods of calculation, and practical applications in investment scenarios.
In an era where information is abundant, discerning investors, financial advisors, and analysts are continually searching for tools to simplify complex data. A solid grasp of averages can lead to more informed choices in investments, market analysis, and financial predictions. By appealing to a diverse range of readersâthose entrenched in finance as well as the casual observerâthis article's aim is to empower you to navigate the waters of financial decisions with confidence.
Investment Dictionaries
Key Terms and Definitions
For many, financial jargon resembles a foreign language. Hence, understanding certain key terms is essential when discussing averages and their applications:
- Mean: The most common average calculated by adding all relevant values and dividing by the total number of values.
- Median: The middle value of a data set when organized in ascending order; it provides a robust measure less sensitive to extreme values.
- Mode: The value that appears most frequently in a data set, useful in determining trends in non-standard distributions.
These terms lay the groundwork for effective communication in the realm of finance. They carry not merely academic weight but practical implications as well.
Practical Applications of Investment Terms
Understanding these concepts is not solely an academic exercise. Here are some scenarios illustrating their applications in real-world contexts:
- Portfolio Analysis: Investors can use means to determine the average returns over a specified period, providing insight into expected future performance.
- Market Trends: The median can help identify whether the majority of stock prices are skewed by outliers, offering a clearer perspective on market behavior.
- Sales Performance: Using mode can inform businesses about the most popular product ranges, shaping inventory and marketing strategies.
By grasping these terms and their implications in your financial endeavors, you're laying the groundwork for smart decision-making.
Expert Advice
Strategic Insights for Different Investment Types
Investors often look for specific strategies based on average calculations. Here are a few considerations depending on your investment type:
- Equities: Analyze historical price means to evaluate if a stock is undervalued or overvalued in the current market.
- Bonds: Use median yield rates to gauge potential returns in comparison to market competition.
- Real Estate: The mode can highlight popular property features, helping investors identify what buyers are seeking in their investments.
Strategizing with these insights allows investors to align their financial moves with market realities, ensuring better positioning in the ever-changing landscape of finance.
Tips for Evaluating Financial Products
Choosing the right financial products can be a daunting task. To simplify this process, consider these tips:
- Research Performance Metrics: Assess the mean returns of investment funds to compare performance against peers.
- Understand the Risks: Utilize median volatility measures to understand potential fluctuations in financial products and tailor your investments to suit your risk tolerance.
- Diversify: Using mode can identify sectors or product types gaining popularity; diversify your portfolio to balance potential risks.
With these considerations, you enhance your ability to choose the best financial tools for your needs, ensuring not only profitability but also sustainability in your strategies.
"In finance, understanding data averages can fundamentally change how an investor approaches their decision-making process."
Understanding Averages
Understanding averages is a fundamental part of grasping more complex statistical concepts. Averages offer a simplified means to summarize data points, cutting through the noise to reveal essential truths about a dataset. Think of averages as a compass that guides analysts and investors alike through the murky waters of numbers - they provide a point of reference that facilitates comprehension and decision-making. When investors consider different financial instruments, for example, they often rely on averages to assess performance and risk.
Moreover, averages help in setting benchmarks. When stakeholders want to gauge performance, such as evaluating a companyâs productivity relative to industry standards, averages become not just useful but essential. In finance, understanding these calculated figures can illuminate trends and help predict future performance. If an investor knows the average return on a particular investment over time, that knowledge serves as context for assessing potential new investments or reallocating current holdings.
The nuances embedded within the concept of averagesâthe choice between mean, median, or modeâcarry significant implications. Thereâs no one-size-fits-all answer. Investors must consider the nature of their data and their specific objectives. After all, averages can tell different stories depending on the dataset and the methods employed. Thus, delving into the specifics will foster a deeper understanding that equips investors to navigate their financial journeys more effectively.
Definition of Average
In its simplest form, an average is a single value that represents a set of numerical values. It serves as a summary statistic that captures essential characteristics of the data. Among the most common types of averages are the mean, median, and modeâeach interpreting data in its unique way.
- Mean is calculated by summing all values and dividing by the count of values.
- Median represents the middle point in a dataset when arranged in order.
- Mode refers to the most frequently occurring value in the dataset.
Understanding these distinctions is crucial, as using the wrong type of average can lead to misleading conclusions.
Importance in Statistics
Averages play a pivotal role in statistics, being fundamental to both descriptive and inferential statistics. They provide a handy method for distilling complex data sets into easily digestible nuggets of information. By summarizing large volumes of data, averages help identify trends and make comparisons.
Furthermore, in the realm of statistical analysis, averages inform hypotheses and guide decision-making. For instance, if a financial analyst notices a consistent increase in the average return of a specific sector, it may prompt further investigation into the sector's health and future prospects.
Importantly, averages allow for a straightforward interpretation of varied data, enabling stakeholders to make quicker, more informed decisions. They make it possible to generate predictions about future performance, based on observed trends. Without such calculations, investors would be flying blind, unable to assess their comparatively risk and reward.
Common Misconceptions
Despite their utility, many misconceptions abound regarding averages. One common misunderstanding is that the average reflects the typical experience of all data points. Itâs crucial to realize that averages can be heavily influenced by outliersâan extreme value can skew the mean to reflect something quite different from the majority of data points.
Another misconception involves equating any average figure with fairness or equality. Just because the average salary in a region might suggest a certain level of prosperity doesnât mean every individual enjoys that prosperity. For example, in a scenario where a handful of high earners skew the mean salary upward, it can create a misleading impression about the overall economic health of the area.
Consequently, one should always look beyond mere averages and examine the underlying data to form a more nuanced understanding. As Albert Einstein famously stated, "Everything should be made as simple as possible, but not simpler." Understanding averages requires a balance between simplification and the complexity inherent in the data being analyzed.
Types of Averages
Understanding the types of averages is essential in the world of data and statistics, especially when one aims to make informed decisions based on numerical data. While it may seem trivial at first glance, the choice of average can significantly influence interpretations and outcomes. This section will explore the three primary types of averages: mean, median, and mode.
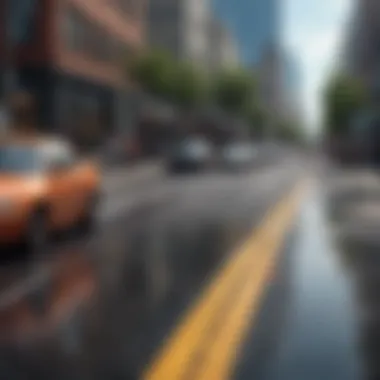
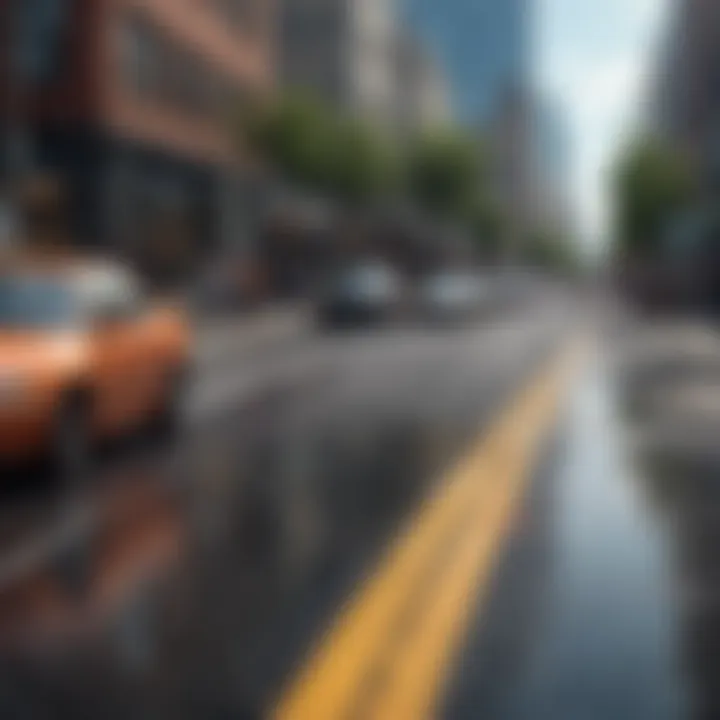
Mean
Definition and Calculation Method
The mean is often called the average in everyday conversation, and it is calculated by adding up a set of values and then dividing by the number of valuesâthis is one of its most attractive features. This straightforward mathematical formulation makes it a go-to choice in many instances.
For example, if you were to find the mean salary of employees in a company, you simply add each employeeâs salary together and divide by the total number of employees. This approach is both efficient and widely recognized, thus making it beneficial for comprehensive analysis in various fields.
However, itâs important to note that while the mean is popular for its simplicity, it can sometimes be misleading, especially in datasets with extreme values. If a few employees earn significantly higher salaries, the mean might not effectively represent the salary situation for the majority.
Examples in Real Life
In the context of everyday scenarios, the mean is frequently applied when evaluating average temperatures, average grades in academic settings, or even average expenditures by consumers during a holiday season. These instances highlight the mean's capacity to provide a quick snapshot of overall trends.
Yet, its utility doesn't come without caveats. In situations laden with veritable outliersâlike calculating the average income of a neighborhood where a few homes are worth millionsâthe mean can distort the picture, suggesting a wealth distribution that doesnât reflect the reality for most.
Influence of Extreme Values
The influence of extreme values on the mean is a critical consideration. If a data set comprises a mix of low and high figures, a few exceptionally high or low values can drag the mean up or down significantly. In essence, it can warp the overall interpretation of data when viewed through the lens of the mean alone.
This sensitivity to extremes renders the mean less reliable in skewed distributions, making it wise to consider complementing it with other measures like median or mode for a more rounded view.
Median
Understanding Median
The median represents the middle point in a dataset when values are arranged in ascending or descending order. This definition sets it apart from the mean, particularly in its resistance to extreme values. In a practical sense, finding the median is often as simple as sorting data and identifying the central number. Itâs frequently regarded as a more reliable indicator of central tendency in skewed distributions.
The unique aspect of the median is its robustness; it remains stable against outliers, thus providing a reliable measure in instances where the mean may fail, especially in financial analysis where the presence of high-value assets can distort the average assessment.
How to Find the Median
To find the median, first, arrange your data in order. If you have an odd number of observations, the median is simply the middle value. On the other hand, if your dataset contains an even number of observations, the median is the average of the two middle numbers. This method is straightforward and easily applicable across various datasets, making it beneficial in both statistical and real-world analyses.
Application in Financial Analysis
In financial analyses, the median serves a critical role, particularly in processes like appraising real estate or evaluating income distributions. Because it isnât affected by extreme values, the median often provides a more accurate representation of what an average participant might expect. Investors should especially value this aspect when considering portfolios, as median returns can shed light on typical performance amidst variable results.
Mode
Definition and Calculation of Mode
The mode identifies the value that appears most frequently in a dataset. This characteristic makes it particularly valuable in understanding repetitive trends or popular choices within a set of data. Unlike the mean and median, the mode can be applied to nominal data, such as survey responses or voting outcomes, broadening its relevance.
In practice, determining the mode involves assessing frequencies. For example, if you conducted a survey on preferred vacation types and "beach" was mentioned most often, it would be classified as your mode. This directness makes the mode appeal to diverse applications in fields ranging from marketing to psychology.
Examples of Mode Usage
Consider a retail environment. A store owner may want to know which product is the best-seller. They can easily identify this by finding the mode of sales data over a specified period. The value of this finding is evident; it assists in inventory management and sales strategies that align with customer preferences.
Despite its advantages, relying solely on mode can lead to oversights. If a product has several variants, knowing merely the most sold doesn't provide the complete picture of overall sales performance, which may include high sales for less popular items as well.
Limitations of Mode
However, a limitation of the mode is that it can sometimes be non-representative in datasets where all values are unique or uniformly distributed. For example, in a case where no number repeats, the mode may not be defined at all or may not reflect any trend. Thus, while mode can provide insightful information, it should not be used in isolation for comprehensive analysis.
"When evaluating datasets, itâs crucial to consider all measures of central tendency to attain a well-rounded understanding of the data."
In summary, knowing how to navigate and apply mean, median, and mode allows one to approach quantitative assessments more effectively, particularly in areas like finance and investments where such measures provide foundational insights.
Calculating the Mean
The mean, often known simply as the average, serves as a cornerstone in statistical analysis. It's the go-to method when one needs to distill a set of data into a single representative value. Understanding how to calculate the mean not only sharpens statistical skills but is also crucial in making informed financial decisions. From assessing salaries to evaluating investment returns, the mean can provide insights that inform strategic choices. However, there are elements to considerâsuch as the impact of outliersâthat can skew results significantly. Thus, mastering this calculation is essential for reliable data interpretation.
Step-by-Step Calculation
Collecting Data
The first step in calculating the mean is collecting data. This process involves gathering all relevant numerical values that will form the basis for your mean calculation. It's imperative to ensure that the data collected is complete and representative of the subject matter. The key characteristic of collecting data lies in its foundation; without accurate and comprehensive data, the mean loses its significance.
Collecting data is popular among analysts, financial advisors, and investors alike because it allows for a detailed overview of financial landscapes. It's unique in its ability to encompass a diverse range of values, offering a nuanced picture of trends, behaviors, and historical performance. However, one downside might be the time required to accurately gather and validate the data, which can sometimes feel like searching for a needle in a haystack in extensive databases.
Summing the Values
Once the data is gathered, the next step is summing the values. This action involves adding all collected numerical entries together to prepare for the mean calculation. The simplicity of summing values is its greatest strengthâit provides a straightforward method for creating the cumulative result which is necessary for finding the mean.
It's essential because it directly influences the computation of the mean; without this step, calculation would be impossible. Despite its appeal, one must remember that summing large datasets requires careful attention to avoid computational errors, which could lead to inaccurate insights. Additionally, in contexts where data types vary significantly, summing might introduce complications that could distort interpretations.
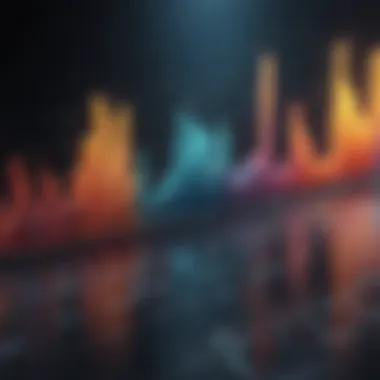
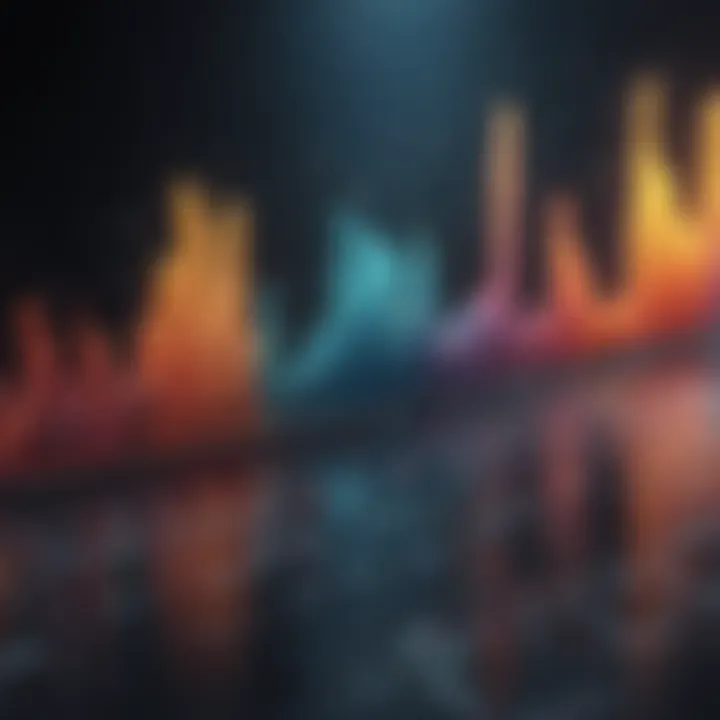
Dividing by the Number of Values
After obtaining the total from summing, youâll arrive at the final step: dividing by the number of values. This is where the total sum is divided by the count of values, yielding the mean. This action solidifies the utility of the prior steps, transforming your aggregated sum into the average we all strive to understand.
Dividing by the number of values is beneficial because it straightforwardly normalizes the total. This step highlights the key characteristic of equidistance: each value contributes equally to the average, thus providing a balanced perspective of your dataset. A potential drawback can be the influence of outliers, where extreme values disproportionately sway the result, therefore necessitating a cautious interpretation of the resultant mean.
Practical Examples
Averages in Salary Data
When it comes to averages in salary data, calculating the mean gives a clear snapshot of compensation structures within organizations. Companies often utilize mean salary calculations to gauge market competitiveness, ensuring they remain attractive to potential employees.
The significance of using averages in salary data is underscored by the ability to draw comparisons across different sectors. By examining the mean salary, your analysis can highlight disparities or trends that might otherwise remain hidden. However, one must tread carefullyâusing the mean without context, especially in industries with wide salary variances, can mislead decision-makers.
Calculating Averages in Investment Returns
Calculating averages in investment returns offers vital insights for both individual and institutional investors. Here, the mean return is frequently referenced to assess performance across portfolios, enabling investors to estimate future risks and rewards based on historical data.
The characteristic of this application is its wealth of information; mean returns help investors identify patterns and performance benchmarks. It aids in making comparative assessments among different funds or stocks. The trade-off comes with the understanding that mean returns can give a deceptively rosy picture in volatile markets, as they may gloss over significant underlying risks associated with outliers or sudden shifts in performance.
Determining the Median
Understanding how to determine the median is crucial for making sense of data that isnât uniformly distributed. The median, as the middle value in a dataset, gives you a clearer picture of the central tendency, especially when outliers might skew the mean. In the realm of finance and investments, accurately determining the median can help in assessing property values or evaluating portfolio risks, ultimately leading to more informed decision-making.
Finding the Median of a Set
Arranging Data
Arranging data is the first step in finding the median. To determine the median, data points must be sorted in numerical order. When the data is arranged, it provides a ladder, allowing you to easily see the middle point. This characteristic of arrangement is not merely a formality; it turns data into a manageable format, ultimately revealing insights that would otherwise remain hidden. An example of why this is important can be seen in the context of stock prices. If you don't arrange them, identifying a potential median value among a sea of numbers can turn chaotic rather quickly, leading to poor analysis.
Identifying the Middle Value
Once the data is arranged, identifying the middle value becomes the next logical task. If there's an odd number of data points, the median is the single middle number. In cases where the dataset contains an even number of entries, the median is calculated by averaging the two central values. This unique feature allows the median to minimize the impact of extreme values, showcasing a true center, unlike the mean which can be heavily affected by outliers. Hence, investors often lean on the median, particularly in volatile markets, where one or two odd figures could distort the average.
Adjustments for Even Sets
Adjustments for even sets are necessary to provide an accurate median when datasets have an even number of entries. As mentioned, the median for an even dataset is determined by averaging the two middle numbers. While this ensures a refined answer, it can sometimes mask underlying variances that may be important in certain analyses. This cyclical nature of calculations, while useful, is essential to keep in mind to avoid over-simplification. For instance, property values in a neighborhood could vary significantly; thus, not considering the range around that median could leave investors vulnerable to misinterpretation of the market value.
Median Applications
The median shines in a couple of key areas, especially within the realms of property and portfolio analysis. Its implementation helps in deriving insights where otherwise the data could mislead.
Use in Property Valuations
In property valuations, using the median price can often give a more accurate view of market trends compared to the average price, which may be distorted by a handful of high or low sales. The median can effectively inform potential buyers about what to expect in a more realistically priced context. For instance, in a neighborhood where a few luxury homes inflate the average price, the median provides a clearer indication of value, ensuring that buyers aren't discouraged by inflated figures. This approach allows for better negotiation and investment decisions, keeping both sellers and buyers on a more even playing field.
Portfolio Analysis
Median analysis in portfolios is also critical. By observing median returns of investment assets over time, investors can gauge overall performance accurately without the clouding influence of any extremely high or low results. This application of the median represents a balance between risk and reward and helps to cultivate a more realistic expectation of returns. Investors can utilize this information to diversify their portfolios smartly and avoid situations where extreme values might misguide their financial strategies.
"The median is a more robust measure of central tendency when dealing with skewed data, providing clarity where the mean may falter."
Using the Mode in Calculations
The mode, often overlooked when discussing averages, serves as a critical tool in various analytical scenarios. Unlike the mean and median, which can sometimes produce distorted views of dataâespecially in skewed distributionsâthe mode zeroes in on the most frequently occurring value, providing specific insights that can drive decision-making. In investment decisions, for example, knowing what the most common sale price for a property is can reveal trends that aren't immediately obvious from other averages.
Determining the Most Frequent Value
Collecting Frequency Data
Collecting frequency data is the foundation for calculating the mode. This process involves tallying each distinct value within a dataset to determine how many times each value appears. It's not simply about collecting numbers; itâs about creating a comprehensive picture of the data landscape. The key characteristic here is simplicity. Mode calculations can be conducted easily without requiring extensive statistical tools, making it a popular choice for quick analyses.
One unique feature of this data collection is its straightforwardness. Unlike processes that may involve complex computations, gathering frequency data just asks you to count occurrences. This makes it particularly beneficial in environments where time is of the essenceâsuch as retail environments where quick decisions can lead to substantial profits. While it doesn't always present the full picture, using it enables analysts to focus on the most relevant trends. Nevertheless, one drawback can be its insensitivity to the spread of data; it will remain a single number while ignoring the additional insights a more comprehensive analysis might offer.
Practical Uses in Retail and Sales Analysis
When it comes to practical applications, understanding the mode can revolutionize retail and sales analysis. By identifying the most frequently purchased products, business owners can tailor their marketing strategies, optimize inventory levels, and ultimately enhance customer satisfaction. The key characteristic of this approach lies in its effectiveness; retailers gain immediate insight into consumer behavior, which can inform inventory decisions without having to delve deep into the entire data set.
A distinct advantage of this method is that it emphasizes popular choices, allowing businesses to align their offerings with customer preferences. Additionally, using mode calculations can help surface trends that sales forecasts might miss, providing a more nuanced view of market dynamics. However, like any analytical method, itâs not without its limitations. The mode does not reflect how sales are distributed across the broader market, and therefore, relying solely on it may lead to decisions that overlook potential impacts from lower-occurring, but still significant, sales.
"Understanding the mode allows businesses to capture nuanced data insightsâpowerful tools in competitive markets that can dictate strategy and performance."
As explored in this section, while the mode might not always be the superstar in the averages ensemble, it plays a vital role when used thoughtfully, especially amidst the bustling world of retail sales. By focusing on the most repeated values, analysts can open doors to new strategies that lead to greater success.
Applications of Averages in Investment Decisions
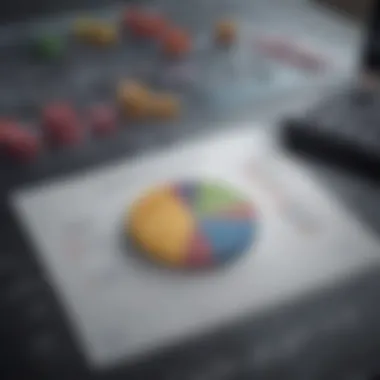
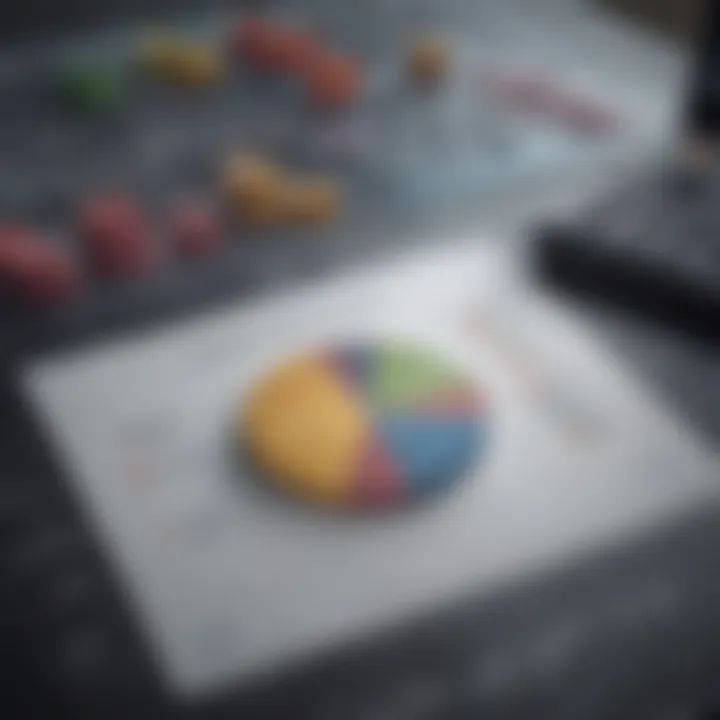
Understanding how averages apply in investment decisions is crucial for investors aiming to navigate the complex financial landscape. Averages help in distilling large datasets into understandable insights, making it easier to evaluate performance and predict outcomes. When deciding where to allocate capital, knowing how to utilize averages can provide a sharper edge.
Comparing Financial Instruments
In the realm of investments, comparing financial instruments using averages offers clarity in uncertainty. By observing the average returns of different assets, investors can gauge which options present better risk-adjusted returns. Such comparisons enable investors to make informed decisions rather than relying on hunches or fleeting market sentiments.
Mean Returns for Risk Assessment
When delving into mean returns, it's essential to recognize how these figures serve as a baseline for assessing risk. Mean returns represent the average profits an asset generates over time. In this article, we highlight why these figures are not just numbers on paper but powerful tools for evaluating potential investments. By looking at historical data of mean returns, an investor can visualize trends and their implications for future performance.
The key characteristic of mean returns lies in its ability to summarize performance succinctly. Investors can quickly spot which assets perform consistently or erratically based on their average returns over specific periods. However, this method isn't without drawbacks; mean returns can be heavily influenced by outliers. For instance, if a few high-performing years skew the average upward, one might mistakenly believe an asset is a low-risk option.
Investor Behavior Insights
Shifting focus to investor behavior, understanding how people tend to react to averages can lend further insights into market dynamics. The average returns guide investor expectations, influencing decisions on whether to buy, hold, or sell assets. This psychological aspect is easily overlooked but vital. Investors often gravitate towards the greener pastures shown by higher averages, disregarding potential risks.
The significant aspect of investor behavior analysis is its reflection of market sentiment. If a majority of investors see positive average returns, they are more likely to buy into these investments, which can create trends. However, such behavior can introduce volatility, especially if the crowd rushes in based on inflated averages. Understanding this can help in predicting market maneuvers and recognizing when to exercise caution.
Analyzing Market Trends
Moving beyond individual investments, averages also play a central role in analyzing overarching market trends. As markets fluctuate, smoothing the data using averages allows analysts and investors to see underlying patterns instead of getting lost in noise.
Smoothing Data Fluctuations
Smoothing is a technique that transforms erratic datasets into comprehensible forms, allowing for easier interpretation. By employing averaging methods, like moving averages, analysts can strip away the daily variations and focus on longer-term trends.
The primary benefit of smoothing data fluctuations is clarity. Investors gain an understanding of the broader market movements without the distractions of minute shifts. However, itâs crucial to remember that smoothing can also obscure short-term opportunities or risks. Thus, while an averaged line may suggest a steady upward trend, sudden shifts could indicate impending volatility.
Predicting Future Movements
Finally, the subject of predicting future movements ties together many threads discussed. Averages help in forecasting market action based on historical data, providing valuable insights into what may happen next. By taking into account various timeframes and leveraging averages, investors can make educated guesses about future performance.
The advantage of using averages here is rooted in historical patternsâwhat has happened before can serve as a guide for what might occur. Yet, while relying on averages facilitates predictions, itâs essential not to become overly reliant on them. Markets are dynamic, subject to myriad external influences not fully captured by past averages.
In summary, the applications of averages in investment decisions showcase the tool's multifaceted utility. By comparing financial instruments, understanding investor behavior, and analyzing market trends, one can navigate the investment landscape with greater confidence.
Limitations of Averages
When discussing averages, itâs crucial to understand their limitations. While calculating mean, median, and mode provides a quick snapshot of a dataset, these figures can sometimes mislead rather than inform. Recognizing the boundaries of these calculations enables more informed decision-making, particularly in financial contexts where precision matters greatly.
Impact of Outliers
One of the most significant concerns with averages is the influence of outliers. An outlier is a value that deviates significantly from the other observations in a dataset. These extreme values can skew the average, leading to potentially misleading interpretations.
For instance, consider a scenario where five investors earn annual returns of 5%, 6%, 7%, 4%, and 100%. The mean return would sway heavily toward that unusual 100% return, coming out at 24.4%. That figure might paint a rosy picture of average performance, but it doesn't reflect the experience of the majority of investors in this situation.
In finance, recognizing the presence of outliers is essential not just for calculations, but for strategy as well. Ignoring those rare exceptional cases can lead to poor investment choices. Investors often talk about these "outliers" as being the "lone wolves" of data, reminiscent of statistical anomalies that skew narratives.
Misinterpretation of Data
Another limitation of averages is the risk of misinterpretation. Averages can boil down complex datasets into terribly simplistic figures. For instance, if a company's average employee salary is reported as $50,000, it might imply that most employees earn relatively near that number. However, if a few executives earn substantially higher salaries, the bulk of the workforce could be earning much less.
This misinterpretation can lead investors or decision-makers to have a skewed understanding of a companyâs financial health or operational efficiency. It's like an iceberg; what you see is only a fraction of the whole â the actual scenario could be underwhelming if you dive deeper.
Moreover, various forms of averages can paint different pictures. The mean could suggest something positive, while the median could reveal a less favorable view â especially in unequal distribution cases. Thus, reading between the lines is vital.
The takeaway is clear: while averages provide valuable insights, they should not be the sole lens through which data is examined. Instead, use them in conjunction with variance, standard deviation, and other statistical measures for a more rounded comprehension of the data landscape.
Understanding the limitations of averages paves the way for better analysis and decision-making. It's a crucial skill for investors, advisors, and analysts alike.
Closure
The significance of the conclusions drawn from previous sections cannot be overstated. Having a solid grasp of averages allows investors, financial advisors, and analysts to make more informed decisions. In a world flooded with data, the ability to analyze this information efficiently is an invaluable skill.
Recap of Key Points
Let's revisit some pivotal takeaways:
- Averages Defined: Mean, median, and mode serve different purposes and have unique applications in analysis.
- Calculation Methods: Understanding how to compute these averages precisely is crucial for accurate financial assessments.
- Applications in Investment: Averaging helps in comparing financial instruments, assessing risks, and analyzing trends, providing insights into market behaviors.
- Limitations Recognized: Awareness of how outliers and potential misinterpretations can distort the data is essential for effective usage.
These points underline the broader implications of averages in financial contexts, enhancing decision-making processes and facilitating strategic planning.
Future Considerations
Looking ahead, several avenues warrant deeper exploration:
- Dynamic Market Conditions: As markets evolve, the methods of calculating and interpreting averages may need to adjust accordingly. Keeping abreast of technological developments could provide new insights into data analytics.
- Integration with Advanced Analytics: Utilizing machine learning and AI in conjunction with averages could improve prediction accuracy, particularly in investment environments.
- Behavioral Economics: Understanding how psychological factors influence the interpretation of averages will enhance investment strategies.
In a sector where precision is key, the future of average calculations holds promises of further sophistication, aiming to harness data more effectively while informing better financial decisions.
"Data is the new oil, and averages are the refining process. The clearer your average, the more reliable your insights."
By advancing our approach to calculating averages, we can better navigate the complexities of financial landscapes, ensuring we harness the information at our disposal to its fullest potential.